本文是seq2seq模型的第一篇,本文根据论文Sutskever, Vinyals and Le (2014)而来
github地址:https://github.com/zhuanxuhit/nd101/blob/master/1.Intro_to_Deep_Learning/11.How_to_Make_a_Language_Translator/1-seq2seq.ipynb
参考的文章:https://github.com/ematvey/tensorflow-seq2seq-tutorials/blob/master/1-seq2seq.ipynb
import helper
看图:
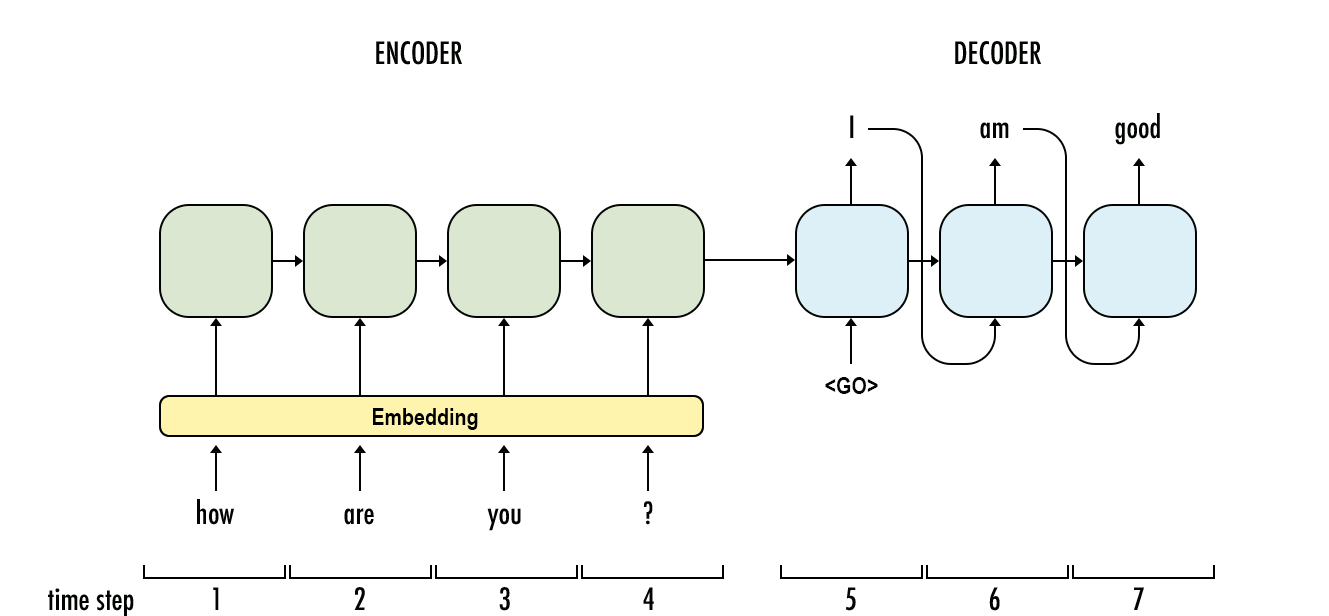
在实际的word2word中,我们会对单词进行embedding操作,此处为了简单起见,我们直接就以数字代表输入了
x = [[5, 7, 8], [6, 3], [3], [1]]
xt, xlen = helper.batch(x)
xt # [max_time_len, batch_size]
array([[5, 6, 3, 1],
[7, 3, 0, 0],
[8, 0, 0, 0]], dtype=int32)
xlen
[3, 2, 1, 1]
在处理中,我们会做一些特殊的处理
- < PAD>: 在训练过程中,batch中每个句子长度会不同了,此时我们对于短的就直接用 < PAD> 来填充的
- < EOS>: EOS代表的句子的结尾
- < UNK>: 对于一些不常见的词汇,直接用UNK替换掉(例如人名)
- < GO>: decode的第一个输入,告诉decode预测开始
定义模型
在定义模型的时候,我们需要确定的是 vocab_size , input_embedding_size 和 encoder_hidden_units 和 decoder_hidden_units ,一旦修改得重新定义模型
import tensorflow as tf
import numpy as np
PAD = 0
EOS = 1
# UNK = 2
# GO = 3
vocab_size = 10
input_embedding_size = 20
encoder_hidden_units = 20
decoder_hidden_units = encoder_hidden_units
对于一个复杂的模型,我们想要去了解他,最好的方式就是看输入和输出,seq2seq的模型其输入和输出是:
- encoder_inputs int32 tensor is shaped [encoder_max_time, batch_size]
- decoder_targets int32 tensor is shaped [decoder_max_time, batch_size]
encoder_inputs = tf.placeholder(shape=(None, None), dtype=tf.int32, name='encoder_inputs')
decoder_targets = tf.placeholder(shape=(None, None), dtype=tf.int32, name='decoder_targets')
我们还需要定义的一个输入是decoder的输入
- decoder_inputs int32 tensor is shaped [decoder_max_time, batch_size]
decoder_inputs = tf.placeholder(shape=(None, None), dtype=tf.int32, name='decoder_inputs')
在模型训练的时候,对于decoder的ouputs我们不会使用,而是直接使用decoder_targets作为decoder的输入,但是在做predictions的时候,我们却会使用decoder的输出作为下一个lstm的输入,这可能会引入 distribution shift from training to prediction.
Embeddings
我们系统的输入encoder_inputs和decoder_inputs都是 [decoder_max_time, batch_size]的形状,但是我们 encoder 和 decoder 的输入形状都是要 [max_time, batch_size, input_embedding_size], 因此我们需要对我们的是输入做一个word embedded
embeddings = tf.Variable(tf.truncated_normal([vocab_size, input_embedding_size], mean=0.0, stddev=0.1), dtype=tf.float32)
encoder_inputs_embedded = tf.nn.embedding_lookup(embeddings, encoder_inputs)
decoder_inputs_embedded = tf.nn.embedding_lookup(embeddings, decoder_inputs)
print(encoder_inputs_embedded)
Tensor("embedding_lookup:0", shape=(?, ?, 20), dtype=float32)
encoder
encoder_cell = tf.contrib.rnn.BasicLSTMCell(encoder_hidden_units)
lstm_layers = 4
cell = tf.contrib.rnn.MultiRNNCell([encoder_cell] * lstm_layers)
# If `time_major == True`, this must be a `Tensor` of shape:
# `[max_time, batch_size, ...]`, or a nested tuple of such
# elements.
encoder_outputs, encoder_final_state = tf.nn.dynamic_rnn(cell,encoder_inputs_embedded,dtype=tf.float32,time_major=True)
del encoder_outputs
此处我们直接删除了 encoder_outputs, 因为在这个场景中我们是不关注的,我们需要的是最后的 encoder_final_state,这又被称为 "thought vector",如果没有引入attention机制,encoder_final_state 就是decoder的唯一输入,用他来作为decoder的init_state来解出decoder_targets。
We hope that backpropagation through time (BPTT) algorithm will tune the model to pass enough information throught the thought vector for correct sequence output decoding.
print(encoder_final_state)
(LSTMStateTuple(c=<tf.Tensor 'rnn/while/Exit_2:0' shape=(?, 20) dtype=float32>, h=<tf.Tensor 'rnn/while/Exit_3:0' shape=(?, 20) dtype=float32>), LSTMStateTuple(c=<tf.Tensor 'rnn/while/Exit_4:0' shape=(?, 20) dtype=float32>, h=<tf.Tensor 'rnn/while/Exit_5:0' shape=(?, 20) dtype=float32>), LSTMStateTuple(c=<tf.Tensor 'rnn/while/Exit_6:0' shape=(?, 20) dtype=float32>, h=<tf.Tensor 'rnn/while/Exit_7:0' shape=(?, 20) dtype=float32>), LSTMStateTuple(c=<tf.Tensor 'rnn/while/Exit_8:0' shape=(?, 20) dtype=float32>, h=<tf.Tensor 'rnn/while/Exit_9:0' shape=(?, 20) dtype=float32>))
decoder
decoder_cell = tf.contrib.rnn.BasicLSTMCell(decoder_hidden_units)
decoder = tf.contrib.rnn.MultiRNNCell([decoder_cell] * lstm_layers)
decoder_outputs, decoder_final_state = tf.nn.dynamic_rnn(
decoder, decoder_inputs_embedded,
initial_state=encoder_final_state,
dtype=tf.float32, time_major=True, scope="plain_decoder",
)
此处我们不关心decoder_inputs,而是关心decoder_outputs,对于decoder_outputs我们加一个fc,active_function=softmax,得到预测的单词
decoder_logits = tf.contrib.layers.fully_connected(decoder_outputs,vocab_size,activation_fn=None,
weights_initializer = tf.truncated_normal_initializer(stddev=0.1),
biases_initializer=tf.zeros_initializer())
# decoder_prediction = tf.argmax(decoder_logits,)
print(decoder_logits)
Tensor("fully_connected/Reshape_1:0", shape=(?, ?, 10), dtype=float32)
decoder_prediction = tf.argmax(decoder_logits,2) # 在这一步我突然意识到了axis的含义。。。表明的竟然是在哪个维度上求 argmax。
print(decoder_prediction)
Tensor("ArgMax:0", shape=(?, ?), dtype=int64)
对于RNN的输出,其shape是:[max_time, batch_size, hidden_units],通过一个FC,将其映射为:[max_time, batch_size, vocab_size]
# learn_rate = tf.placeholder(tf.float32)
stepwise_cross_entropy = tf.nn.softmax_cross_entropy_with_logits(
labels=tf.one_hot(decoder_targets, depth=vocab_size, dtype=tf.float32),
logits=decoder_logits,
)
loss = tf.reduce_mean(stepwise_cross_entropy)
train_op = tf.train.AdamOptimizer().minimize(loss)
试运行
deep learning is a game of shapes
当我们build graph的时候,如果shape错误就马上会提示,但是一些其他的shape检查,只有我们运行的时候才会发现错误
with tf.Session() as sess:
sess.run(tf.global_variables_initializer())
batch_ = [[6], [3, 4], [9, 8, 7]]
batch_, batch_length_ = helper.batch(batch_)
print('batch_encoded:\n' + str(batch_))
din_, dlen_ = helper.batch(np.ones(shape=(3, 1), dtype=np.int32),
max_sequence_length=4)
print('decoder inputs:\n' + str(din_))
pred_ = sess.run(decoder_prediction,
feed_dict={
encoder_inputs: batch_,
decoder_inputs: din_,
# learn_rate:0.1,
})
print('decoder predictions:\n' + str(pred_))
print("build graph ok!")
batch_encoded:
[[6 3 9]
[0 4 8]
[0 0 7]]
decoder inputs:
[[1 1 1]
[0 0 0]
[0 0 0]
[0 0 0]]
decoder predictions:
[[9 6 6]
[9 6 2]
[9 6 2]
[9 9 4]]
build graph ok!
模拟训练
我们为了简单起见,产生了随机的输入序列,然后decoder原模原样的输出
batch_size = 100
batches = helper.random_sequences(length_from=3, length_to=8,
vocab_lower=2, vocab_upper=10,
batch_size=batch_size)
print('head of the batch:')
for seq in next(batches)[:10]:
print(seq)
head of the batch:
[7, 2, 9, 2, 2, 4, 4]
[6, 9, 8, 5, 2, 3]
[9, 3, 2, 4, 7]
[2, 5, 3, 3, 6, 8, 9]
[2, 4, 8, 5, 5, 3]
[2, 6, 3]
[3, 5, 2, 2]
[9, 5, 3]
[8, 5, 4, 2]
[4, 9, 5, 2, 4, 9]
def next_feed():
batch = next(batches)
encoder_inputs_, _ = helper.batch(batch)
decoder_targets_, _ = helper.batch(
[(sequence) + [EOS] for sequence in batch]
)
decoder_inputs_, _ = helper.batch(
[[EOS] + (sequence) for sequence in batch]
)
return {
encoder_inputs: encoder_inputs_,
decoder_inputs: decoder_inputs_,
decoder_targets: decoder_targets_,
}
当encoder_inputs 是[5, 6, 7]是decoder_targets是 [5, 6, 7, 1],1代表的是EOF,decoder_inputs则是 [1, 5, 6, 7]
loss_track = []
max_batches = 3001
batches_in_epoch = 1000
with tf.Session() as sess:
sess.run(tf.global_variables_initializer())
try:
for batch in range(max_batches):
fd = next_feed()
_, l = sess.run([train_op, loss], fd)
loss_track.append(l)
if batch == 0 or batch % batches_in_epoch == 0:
print('batch {}'.format(batch))
print(' minibatch loss: {}'.format(sess.run(loss, fd)))
predict_ = sess.run(decoder_prediction, fd)
for i, (inp, pred) in enumerate(zip(fd[encoder_inputs].T, predict_.T)):
print(' sample {}:'.format(i + 1))
print(' input > {}'.format(inp))
print(' predicted > {}'.format(pred))
if i >= 2:
break
print()
except KeyboardInterrupt:
print('training interrupted')
batch 0
minibatch loss: 2.301229476928711
sample 1:
input > [4 8 3 3 4 8 0 0]
predicted > [0 0 0 0 0 0 0 0 0]
sample 2:
input > [4 8 7 8 4 3 0 0]
predicted > [0 0 0 0 0 0 0 0 0]
sample 3:
input > [6 4 3 0 0 0 0 0]
predicted > [6 0 0 0 0 0 0 0 0]
batch 1000
minibatch loss: 0.958212673664093
sample 1:
input > [7 2 6 8 0 0 0 0]
predicted > [7 7 3 3 1 0 0 0 0]
sample 2:
input > [2 6 8 6 3 8 0 0]
predicted > [3 3 6 6 6 6 1 0 0]
sample 3:
input > [5 2 4 4 0 0 0 0]
predicted > [5 4 4 4 1 0 0 0 0]
batch 2000
minibatch loss: 0.3982703983783722
sample 1:
input > [8 7 8 0 0 0 0 0]
predicted > [8 7 8 1 0 0 0 0 0]
sample 2:
input > [3 7 9 5 3 0 0 0]
predicted > [3 7 8 5 9 1 0 0 0]
sample 3:
input > [2 8 9 2 0 0 0 0]
predicted > [2 3 9 2 1 0 0 0 0]
batch 3000
minibatch loss: 0.27779871225357056
sample 1:
input > [3 4 5 4 3 8 4 0]
predicted > [3 4 4 5 3 2 4 1 0]
sample 2:
input > [5 4 6 3 8 0 0 0]
predicted > [5 4 6 3 2 1 0 0 0]
sample 3:
input > [4 7 6 0 0 0 0 0]
predicted > [4 7 6 1 0 0 0 0 0]
%matplotlib inline
import matplotlib.pyplot as plt
plt.plot(loss_track)
print('loss {:.4f} after {} examples (batch_size={})'.format(loss_track[-1], len(loss_track)*batch_size, batch_size))
loss 0.2582 after 300100 examples (batch_size=100)